The Comprehensive Guide To IDF: Understanding Its Importance And Applications
In the realm of data management and analysis, the term "IDF" plays a crucial role in how we interpret and process information. This article will delve deep into the concept of IDF, exploring its definition, significance, and applications across various fields. We aim to provide you with a thorough understanding of IDF, its components, and how it integrates with other data metrics to enhance decision-making processes.
As we navigate through this guide, you will discover the intricacies of IDF, its mathematical foundations, and its practical implications in the modern data landscape. We will also highlight its relevance in industries such as marketing, information retrieval, and data science, emphasizing why a solid grasp of IDF is essential for professionals in these fields.
By the end of this article, you will have a comprehensive understanding of IDF, armed with knowledge that can enhance your expertise and decision-making in your respective domain. Let’s embark on this enlightening journey into the world of IDF.
Table of Contents
- What is IDF?
- Importance of IDF
- Mathematical Foundation of IDF
- Applications of IDF
- IDF in Marketing
- IDF in Data Science
- IDF in Information Retrieval
- Future of IDF
What is IDF?
IDF stands for Inverse Document Frequency. It is a metric used in information retrieval and text mining that quantifies how much information a word provides, based on its frequency across a set of documents. The core idea behind IDF is that terms that are common across many documents provide less informative value than those that appear in fewer documents.
To better understand IDF, it is important to look at it in conjunction with TF (Term Frequency). While TF measures how frequently a term appears in a document, IDF assesses the importance of the term in the overall corpus. The combination of TF and IDF is widely used in algorithms such as TF-IDF, which helps in ranking the relevance of documents in response to a search query.
Importance of IDF
IDF is crucial for several reasons:
- Enhances Relevance: By assessing the uniqueness of terms, IDF helps in filtering out less relevant information.
- Improves Search Algorithms: Search engines use IDF to deliver more accurate results to user queries.
- Facilitates Better Data Analysis: In data science, IDF aids in feature selection, allowing analysts to focus on significant variables.
Understanding IDF in Context
IDF can be particularly useful in contexts where the volume of data is large and diverse. For instance, in large-scale text analysis, utilizing IDF can significantly improve the quality of insights derived from the data.
Mathematical Foundation of IDF
The formula for calculating IDF is relatively straightforward:
IDF(t) = log(N / df(t))
Where:
- IDF(t): The Inverse Document Frequency of term t.
- N: The total number of documents in the corpus.
- df(t): The number of documents containing the term t.
This formula highlights that as the number of documents containing a term increases, the IDF value decreases, indicating that the term is less informative. Conversely, terms that are present in fewer documents will have a higher IDF value, thus indicating greater significance.
Applications of IDF
IDF has a wide range of applications across various domains:
- Text Mining: IDF is used to identify key terms that can help in summarizing documents.
- Search Engine Optimization (SEO): Understanding IDF can enhance keyword strategies for better visibility.
- Machine Learning: IDF is utilized in feature engineering, aiding in the development of predictive models.
IDF in Marketing
In the marketing realm, IDF plays a significant role in optimizing content for search engines. By understanding which keywords have higher IDF values, marketers can tailor their content to target specific audiences more effectively. This not only improves search rankings but also enhances user engagement by providing relevant information.
Some strategies marketers can employ using IDF include:
- Keyword Research: Identifying high-IDF keywords can help in creating targeted marketing campaigns.
- Content Creation: Developing content that focuses on niche topics with higher IDF can attract a more dedicated audience.
- Competitive Analysis: Analyzing competitors’ keyword strategies using IDF can reveal opportunities for differentiation.
IDF in Data Science
For data scientists, IDF is an essential tool in natural language processing (NLP) tasks. It helps in feature selection and dimensionality reduction by identifying relevant features in a dataset. This results in more efficient models that are easier to interpret and deploy.
In data science projects, IDF can be applied in various ways:
- Text Classification: Using IDF to select significant terms can improve classification accuracy.
- Sentiment Analysis: Identifying impactful words using IDF can enhance sentiment detection models.
- Recommendation Systems: IDF can be integrated into recommendation algorithms to provide more personalized experiences.
IDF in Information Retrieval
IDF is foundational in information retrieval systems, particularly in search engines. By utilizing IDF in conjunction with TF, search engines can rank documents based on their relevance to a user's query. This ensures that users receive the most pertinent information quickly and efficiently.
Key aspects of IDF in information retrieval include:
- Document Ranking: IDF helps in ranking documents based on their informational content.
- Query Expansion: IDF can assist in expanding search queries to include related terms, improving retrieval performance.
- Precision and Recall: IDF plays a role in enhancing the precision and recall metrics of search systems.
Future of IDF
The future of IDF looks promising as data continues to grow exponentially. With advancements in machine learning and artificial intelligence, the methods of calculating and applying IDF are likely to evolve further. This may lead to more refined techniques that better account for the nuances of language and context.
As industries increasingly rely on data-driven decision-making, understanding and utilizing IDF will remain a critical skill for professionals in various fields. Staying abreast of developments in IDF and its applications can provide a competitive edge in the job market.
Conclusion
In summary, IDF is a powerful metric that significantly enhances our understanding of information retrieval and data analysis. Its applications across marketing, data science, and information retrieval highlight its importance in the modern data landscape. By leveraging IDF, professionals can improve their decision-making processes and deliver more relevant insights.
We encourage you to explore IDF further and consider how it can be applied in your field. Leave a comment below if you have questions or insights regarding IDF, and feel free to share this article with your network!
Final Thoughts
Thank you for taking the time to read this comprehensive guide on IDF. We hope you found it informative and engaging. Be sure to return to our site for more articles that delve into the fascinating world of data science and information management.
Patrick Cantlay: A Rising Star In Professional Golf
Crosby Raiders: A Comprehensive Overview Of The Football Club
Ayman Mohyeldin: A Comprehensive Look At The Acclaimed Journalist
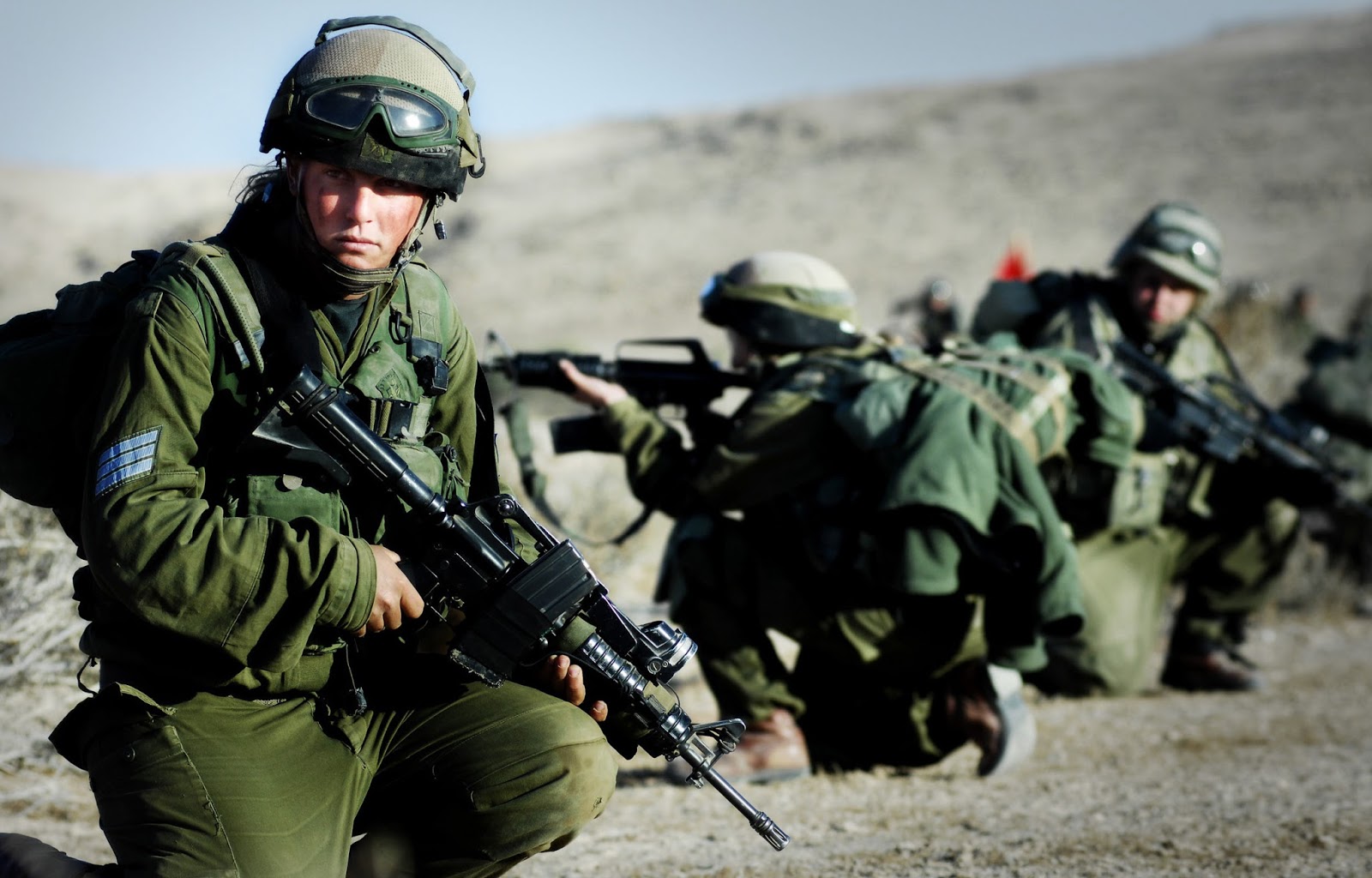

